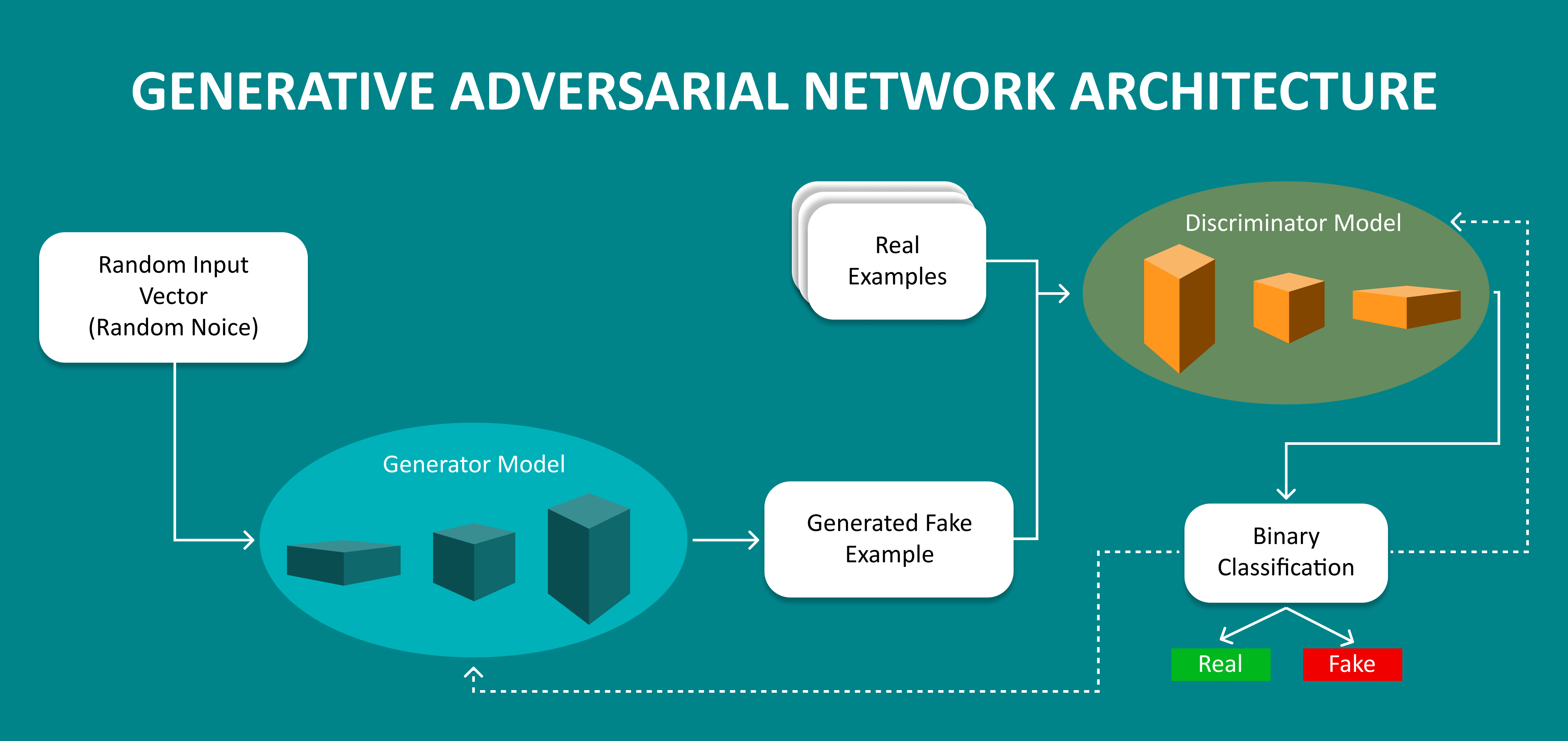
Welcome to the world of AI! AI is advancing quickly and pushing the limits of what machines can do. You've probably seen AI do things like recognizing faces in photos, playing games like chess, and even creating simple music.
But what if AI could do more than just analyze data? What if it could create entirely new and original things?
That's where Generative AI comes in. It's a powerful technology that doesn't just analyze data, but also creates brand new information. You can see examples of what it can do in our blog post "Generative AI: Real-like content produced by AI".
In this blog, we'll explore the different techniques behind Generative AI and how it's changing the game across various industries. Imagine AI not just analyzing data but actively shaping the Future of AI in Industries. This is the exciting potential of Generative AI. We'll delve into these techniques and see how they're being applied, including the different types of generative AI.
Generative AI involves various techniques, each with its strengths and applications. Let's explore some of the most prominent generative AI models behind this groundbreaking technology:
Imagine two AI artists competing in an artistic duel. That's the concept behind GANs! They consist of two neural networks:
Through this competition, the generator improves at creating realistic outputs, while the discriminator becomes better at identifying fakes. This adversarial process leads to high-quality outputs, making GANs perfect for tasks like generating lifelike images, creating new music genres, or even composing realistic dialogue.
However, GANs can be challenging to train and may sometimes produce nonsensical outputs. Achieving highly detailed or complex outputs can also be difficult.
Unlike the competitive nature of GANs, VAEs take a collaborative approach. They consist of two parts:
VAEs are excellent at learning latent representations of data, useful for tasks like dimensionality reduction and anomaly detection. They are also good at generating new data that is consistent and smooth. However, they may struggle with highly complex or detailed outputs.
These models generate data methodically, building it one piece at a time, using previously generated elements to inform the next one. This can be achieved through techniques such as:
Autoregressive models are known for their ability to produce high-quality outputs, especially for tasks like generating realistic and coherent text, composing music, or writing computer code. However, they can be slower compared to other generative models.
Diffusion models take a unique approach to generation. They start with a real data sample and gradually add noise to it until it becomes completely random. Then, they learn to reverse this process, starting with random noise and progressively denoising it to recover the original data or create entirely new variations of it.
This method makes diffusion models excellent at generating complex data like images. They can capture intricate details and produce highly realistic outputs.
This approach leverages reinforcement learning, where an agent interacts with an environment and learns through trial and error. In generative AI, the agent could be a generative model, and the environment could be a dataset of real data. The agent receives rewards for generating data similar to the real data in the environment.
Reinforcement learning offers a promising approach for training generative models with specific goals. By rewarding desired outputs during training, the model can be fine-tuned to generate data that meets specific criteria. While still under development, reinforcement learning holds significant potential for shaping the future of Generative AI for business.
Generative AI is reshaping various fields:
Generative AI is shaping our world, personalizing education, entertainment, and movie effects. As it advances, expect even more changes in various industries and daily life.
Generative AI is revolutionizing creative industries by:
Generative AI is rapidly evolving, with researchers exploring new frontiers and refining existing techniques. Here's what the future might hold:
1. Enhanced Control and Explainability: Researchers are developing generative models that offer more control over the generation process. Users will be able to fine-tune outputs to match specific goals or preferences.
2. Multimodal Generation: Future AI systems may combine different modalities, like text and image generation. Imagine AI that not only creates realistic pictures but also generates accompanying narratives or captions.
3. Human-in-the-Loop AI: We might see a collaborative approach where humans and generative AI work together. AI models can generate creative ideas, while humans provide direction or refine outputs to achieve the desired outcome.
By responsibly developing generative AI, we can ensure it reaches its full potential.
Generative AI, including GANs, VAEs, and autoregressive models, offers unique strengths. It revitalizes creative industries and accelerates scientific discoveries. However, its societal role must be carefully considered. The future of generative AI depends on both technological advancements and ethical use. By harnessing its potential for good, we can ensure a brighter future for all.
We, at Seaflux, are AI undefined Machine Learning enthusiasts, who are helping enterprises worldwide. Have a query or want to discuss AI projects where generative AI can be leveraged? Schedule a meeting with us here, we'll be happy to talk to you.
Business Development Executive