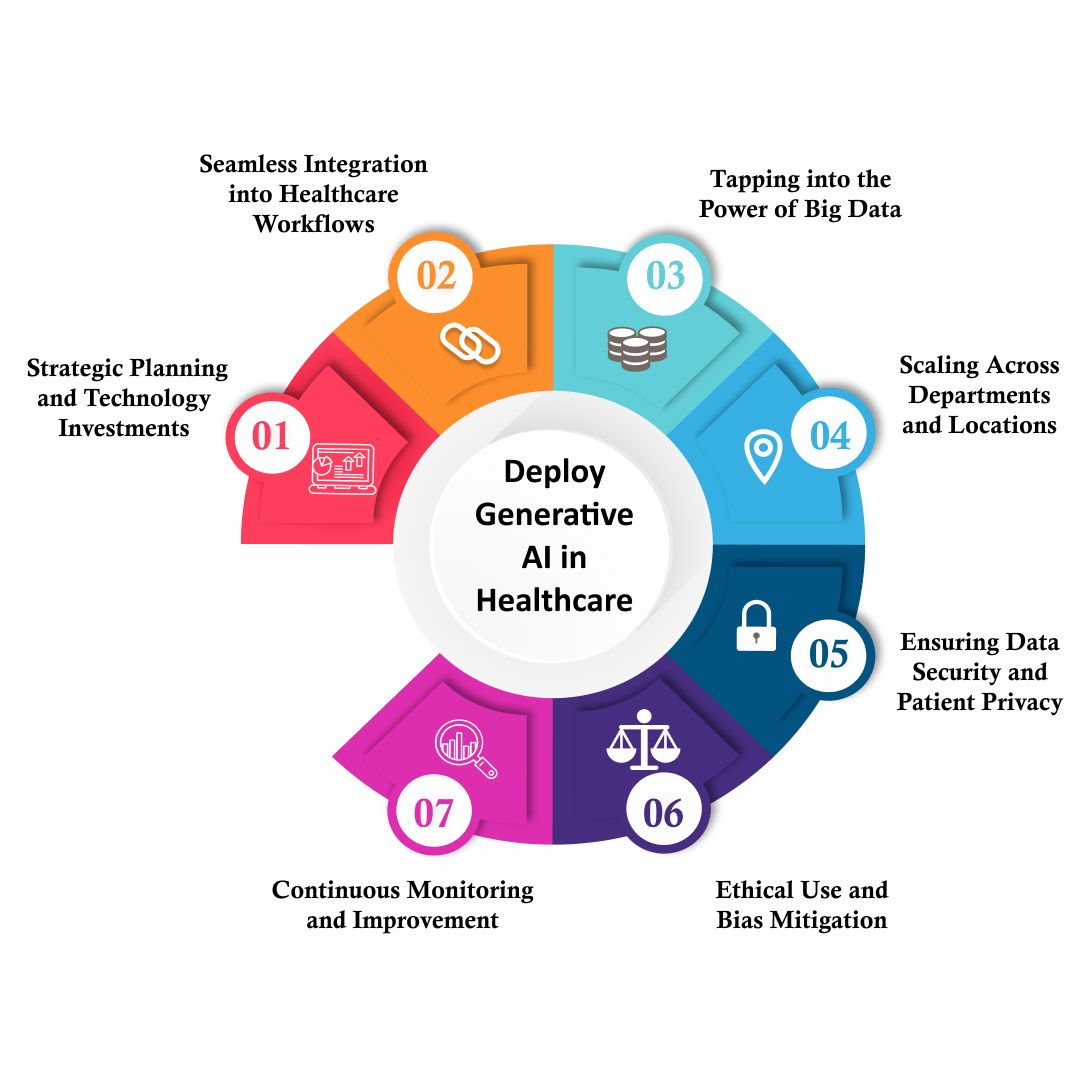
Generative AI is a transformative technology that is changing multiple sectors, and the healthcare sector is no exception. By producing new data based on existing input data, generative AI for healthcare is improving patient outcomes, medical science, diagnostics, and healthcare management. In this blog, we will discuss how Generative AI is changing the healthcare sector, key applications, challenges, and opportunities.
Generative AI is the term given to the algorithm that creates new information based on patterns learned from existing data. Unlike traditional AI, which only processes and analyzes data because it exists, generative AI produces new data points, predictions, and simulations. In medicine, this can translate to creating new treatment protocols, creating realistic patient models, simulating the development of the disease, and even predicting medical results.
Liberal AI's ability to induce innovation in the AI in healthcare industry is endless, from patient results to operational efficiency. Since health services are changing in the direction of becoming rapidly data-driven, the contribution from generative AI healthcare innovations will be important to promote both research and practice, alongside the growing role of machine learning in healthcare. AI healthcare startups are also emerging rapidly to capitalize on this transformative shift, offering specialized solutions to long-standing challenges. Similarly, AI development companies are playing a key role in building scalable platforms that support these innovations across the healthcare ecosystem.
Generative AI is already proving its worth in various applications across healthcare. Let's explore some notable AI in healthcare examples and how they are revolutionizing the industry.
Examples: Oncologists could utilize an AI tool to provide a personalized cancer therapy plan based on how a patient may have responded to a prior therapy and their genetic structure.
Example: AI-based systems such as Zebra Medical Vision change radiology by inspecting medical images and informing doctors about potential abnormalities.
Examples: Insilico Medicine used generic AI to construct new drug molecules, and reduced the time to detect treatment for disease conditions such as cancer and fibrosis.
Example: undefineda class="code-link" href="https://www.mobihealthnews.com/news/babylons-ai-enabled-symptom-checker-added-recently-acquired-higis-app" target="_blank"undefinedBabylon Health's AI-based chatbotundefined/aundefined
provides customers with non-public clinical history- and symptom-led consultations as a handy first touch factor for healthcare.
Example: Hospitals can use AI solutions to predict ICU bed requirements during flu season or to analyze data to figure out the correct staffing levels.
Enterprises should begin with a well-defined strategy that clearly emphasizes what they want to achieve with Generative-AI. Whether there is an increase in clinical accuracy, adapting patient aids, predicting the outbreak of the disease, or accelerating the development of the drug, the goal should be specific and measurable.
For example, a hospital network might aim to use Gen-AI for early detection of heart diseases based on patient records and wearable device data.
At the same time, investing in undefineda class="code-link" href="https://www.seaflux.tech/portfolio/cancer-diagnostics-solution" target="_blank"undefinedrobust infrastructure like cloud computingundefined/aundefined
, secure data storage, and healthcare-specialized AI platforms is essential to support these ambitions.
For Gen-AI to deliver value, it must fit naturally into existing clinical and administrative workflows.
This is important not only to handle technical integration with Electronic Health Records (EHR) systems, but also to ensure that health professionals are trained and feel safe using these AI units.
Imagine an oncology department that adopts an AI system suggesting individual chemotherapy plans, doctors will require both technical support and clinical guidance when interpreting AI recommendations.
The actual power of generative AI is that it can handle and learn from huge amounts of data.
Healthcare organizations need to develop trustworthy pipelines for the collection, cleansing, and processing of data from multiple sources such as EHRs, radiology images, lab results, and genomic databases.
For example, a big healthcare organization may amalgamate imaging information from different branches to train an AI that can detect early cancers that would otherwise go undetected.
Once Gen-AI tools show success in one department, they can be scaled across other units, clinics, or even across different types of healthcare facilities.
Scaling requires flexible technology, modular AI models, and an organizational culture ready to adapt.
For example, a mental health clinic that uses Gen-AI to predict patient no-shows might extend this system across other specialties like cardiology or dermatology, adjusting the models as needed.
When the gen-AI tools show success in a department, they can also score in other devices, clinics, or different types of health facilities.
Scaling requires flexible techniques, modular AI models, and an organizational culture designed for adaptation.
For example, a mental health clinic that uses gen-AI to predict the patient the NO-show, may expand this system to other specialties such as cardiology or dermatology, and adjust the model as needed.
Generative AI systems can inadvertently reflect the bias in either their architecture or their training data.
Companies need frameworks to organize how we will observe AI behaviour, audit outcomes related to equity, and stave off the possibility of discrimination on the basis of race, sex, or level of social and economic risk.
For example, if a diagnostic tool shows a bias toward underdiagnosing specific groups, there has to be a timely response from healthcare providers.
Deploying generative AI in healthcare is not a one-time project; it’s an ongoing process. Companies should install feedback loops where AI performance is regularly reviewed, analyzes the real-world results, and the system is updated as medical knowledge and clinical guidelines to developed.
An example can update a gene-AI model annually because new treatment protocols for diabetes or cancer are available.
Generative AI in healthcare is expected to drive substantial growth in the coming years. According to market research According to market research, undefineda class="code-link" href="https://www.xenonstack.com/blog/agentic-ai-healthcare-system"_blank"undefinedAgentic AIundefined/aundefined
in healthcare is poised for substantial growth, with projections estimating a CAGR of 35.14% from 2023 to 2032, surpassing $21.74 billion by 2032. These trends clearly reflect the dynamic potential of AI in healthcare industry developments. AI healthcare startups will likely play a significant role in shaping this future by driving niche innovations and partnering with traditional healthcare providers to bring cutting-edge solutions to market faster.
Here are some additional insights into the future of AI in healthcare:
undefineda class="code-link" href="https://www.seaflux.tech/portfolio/aws-healthcare-consultation-platform" target="_blank"undefinedTelehealthundefined/aundefined
is expected to unfold with the advent of real-time monitoring via teleporting monitoring systems. AI will provide insights from the data gathered while remote monitoring to enable encourage those healthcare providers to continue the conversation with patients and severely optimise it as an option for chronic care management for patients who don’t leave the house as frequently or at all, which can add a lot of value for patients overall.Generative AI is transforming healthcare by presenting new ways of addressing several traditional challenges of healthcare; heightened diagnostic capacity, individualized therapy, increased efficiency of operations, and objective drug discovery will reshape the healthcare ecosystem profoundly. Providers are faced with a hurdle of addressing issues of ownership, bias, and regulatory impediments to take the full leverage of AI in healthcare industry. As the healthcare sector changes and adopts innovation, generative AI and machine learning in healthcare will lead the discourse and assist in enhancing the quality of patient care.
Seaflux Technologies is a leading Healthcare App Development company that enables healthcare companies to take advantage of new technology by providing custom healthcare solutions, affordable solutions, and programmable, secure digital health services, all to enhance patient care and operational efficiency. We are an end-to-end digital transformation service provider offering new digital experiences, advanced AI, undefineda class="code-link" href="https://www.seaflux.tech/industry/healthcare" target="_blank"undefinedhealthcare AI solutionsundefined/aundefined
, data analytics, undefineda class="code-link" href="https://www.seaflux.tech/blockchain-development-services" target="_blank"undefinedblockchainundefined/aundefined
, undefineda class="code-link" href="https://www.seaflux.tech/hire-devops" target="_blank"undefinedDevOpsundefined/aundefined
, undefineda class="code-link" href="https://www.seaflux.tech/cloud-computing-services/cloud-automation" target="_blank"undefinedcloud computingundefined/aundefined
and undefineda class="code-link" href="https://www.seaflux.tech/custom-software-development" target="_blank"undefinedcustom healthcare software development servicesundefined/aundefined
that will disrupt all types of operations and transform obstacles into digital experiences. Our agile methodology, rigorous development cycles, and industry understanding drive innovation, growth, and real results for our clients that help them compete in an ever-changing digital evolution environment.
undefineda class="code-link" href="https://calendly.com/seaflux/meeting?month=2025-04" target="_blank"undefinedSchedule a Meetingundefined/aundefined
with us today to explore how Seaflux Technologies can help your organization leverage the power of generative AI and more!
Marketing Executive